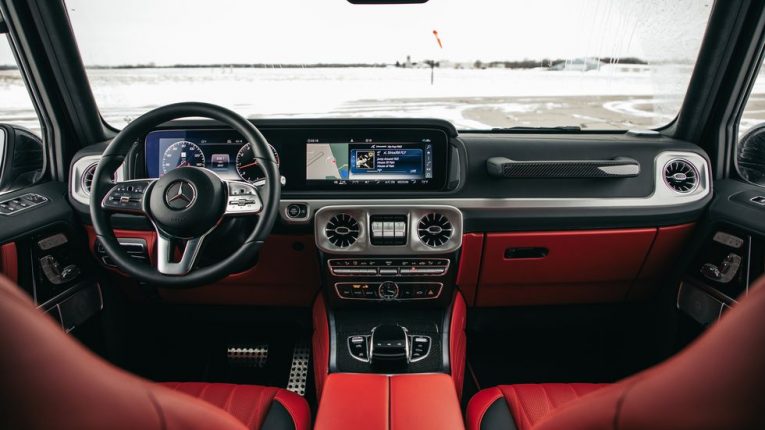
Can Machine Learning Predict Car Accident Risk?
The United States is among the leading countries in road traffic. There are close to 280 million vehicles on the roads and more than 227 million American drivers. These conditions also give rise to high accident numbers. In 2019 more than 30,000 people died in over 33,000 car crashes across the U.S.
In this context road safety is a crucial concern for many experts. They are constantly on the lookout for new strategies to help increase safety measures. One such innovative approach is using machine learning to predict car accident risk. This preventative measure can serve as a warning system to the public and improve overall road safety.
In the following, we will take a closer look at three different models used by scientists. These studies show how machine learning can be used to predict real-time car crashes.
Real-Time Traffic Accident Prediction with the Deep Neural-Network Model
In a study about car accidents in the U.S., scientists used a deep neural-network model on sparse data to predict real-time accident risk. Researchers named the model Deep Accident Prediction (DAP).
They have set off to address some of the shortcomings of existing literature on the topic, namely: dependence on vast data sets, little or no relevance for real-time risk prevention, and relying on data that is not easily available to other researchers. To achieve this, researchers relied on the following main attributes:
- Time
- Weather
- Points-of-interest
- Traffic events
The research obtained satisfactory results, especially when compared to other existing models. They highlighted the importance of time, traffic information, and points-of-interest on real-time accident prediction. What is more, they created a country-wide data set that they made publicly available, thus helping other researchers in their quest to predict and prevent traffic accidents.
The focus for the data analysis fell on six cities: Atlanta, Austin, Charlotte, Dallas, Houston, and Los Angeles. A studyfound that the most dangerous roads in the US are located in the South. Georgia, for example, ranked 14th on the list, with 15.1 per 100,000 deaths in traffic accidents. When involved in a crash, it is advised to contact an auto accident lawyer in Atlanta to help with the proceedings that follow.
Decision Tree Method
Another real-time prediction model for traffic accidents comes from a study on urban expressways in China. Researchers have built their data set using traffic sensors across two years. For the analysis, they have used information up to 30 minutes before the crash. The data was examined through the adaptive neural network fuzzy inference system (ANFIS) and the decision tree method.
Decision trees are also known as classification trees because they are used to classify data, and the results can be viewed in a tree structure. This statistical tool stems from data mining and machine learning. It was quickly adopted in many fields because it is relatively easy to work with, and it is nonparametric.
Researchers have used the mixed method to “train and establish the real-time crash risk prediction model.” Prediction accuracy was as high as 65%.
The Improved Deep Learning Model
In a 2018 study on the prediction of traffic crashes, scientists have successfully implemented a deep learning model into their analysis. Previous machine learning had certain limitations in working with raw data, but deep learning overcomes this obstacle. The computational models used can learn different representations of data.
This group of researchers has focused on the interaction between the following components: traffic, environment, roadways, and traffic accidents. They have selected Knox County from Tennessee to test their model. Results show that they are on to a viable model that can significantly improve existing traffic crash predictions.
Final Thoughts
Car accidents are a common occurrence across the U.S. that lead to many fatalities. Agencies, researchers, and policymakers are in a rush to help prevent such accidents and to help decrease response time when a car crash does occur.
Scientists have relied on machine learning to analyze the interaction between different elements (e.g. road condition, traffic, weather) and traffic incidents. The results can serve authorities in setting up better countermeasures and make roads safer.